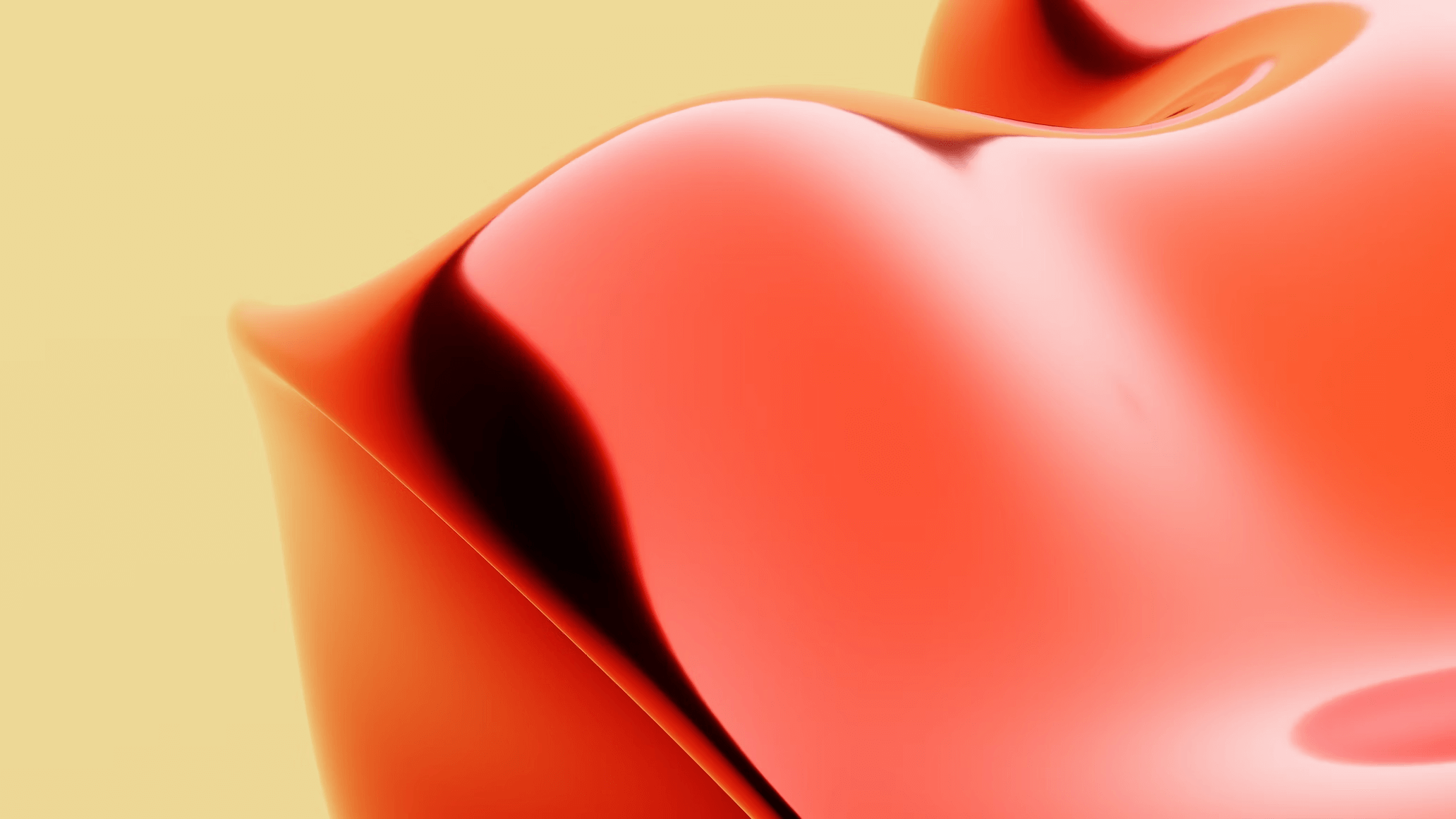
Introduction to Machine Learning and its Applications
Machine learning is revolutionizing the financial landscape. As technology advances, its ability to analyze vast amounts of data has opened new doors for predicting fund performance. Imagine a world where algorithms can sift through miles of market trends and economic indicators in seconds, providing insights that were once only achievable through extensive research and intuition.
The potential applications are immense, especially in fund prediction. Investors now have access to tools that can enhance decision-making processes and improve returns on investments. This convergence of finance and artificial intelligence is reshaping how we understand market dynamics.
Are you ready to explore how machine learning brings smart predictions into the realm of funds? Let’s dive deep into this fascinating intersection of technology and finance!
The Benefits of Using Machine Learning in Fund Prediction
Machine learning offers transformative benefits in fund prediction, enhancing accuracy and efficiency. By analyzing vast datasets, these algorithms identify patterns that are often invisible to human analysts.
Speed is a significant advantage. Machine learning models process information rapidly, allowing for real-time predictions. This agility can lead to better decision-making in fast-paced financial markets.
Additionally, machine learning reduces the impact of human biases. Algorithms rely on data rather than emotions or preconceived notions, resulting in more objective outcomes.
Scalability also plays a role; as the volume of data grows, machine learning systems adapt without requiring extensive manual intervention. They learn continuously from new information, refining their predictions over time.
Moreover, these technologies offer personalized insights tailored to individual investor profiles. Users can receive customized recommendations based on their risk tolerance and investment goals—creating a smarter approach to fund management.
Different Types of Machine Learning Techniques used in Fund Prediction
Machine learning employs various techniques tailored for fund prediction. Supervised learning stands out, where algorithms learn from labeled datasets. This method trains models to recognize patterns, helping predict future trends based on historical data.
Unsupervised learning is another technique used in this field. It identifies hidden structures in unlabeled data. By discovering these patterns, investors can gain insights into market dynamics without predefined outcomes.
Reinforcement learning offers a dynamic approach by adapting strategies through trial and error. This technique continually refines predictions based on feedback from the environment.
Additionally, ensemble methods combine multiple models to improve accuracy. Techniques like bagging and boosting leverage diverse algorithms for robust predictions.
Each of these machine learning techniques contributes uniquely to fund prediction, enhancing decision-making processes and investment strategies.
Challenges and Limitations of Machine Learning in Fund Prediction
Machine learning offers incredible potential for fund prediction, yet it is not without its challenges. One significant hurdle is the quality of data. Inaccurate or incomplete datasets can lead to misleading predictions.
Another challenge lies in model complexity. Many algorithms require careful tuning and expertise to optimize performance. A poorly tuned model may fail to capture important market trends.
Additionally, machine learning models can suffer from overfitting. This occurs when a model learns noise rather than meaningful patterns, which diminishes its effectiveness in real-world scenarios.
The fast-paced nature of financial markets also poses difficulties. Algorithms trained on historical data may struggle to adapt quickly to sudden changes or unforeseen events.
Transparency remains an issue. The “black box” nature of some algorithms makes it hard for stakeholders to understand how decisions are made, raising concerns about accountability and trustworthiness in fund management.
Real-life Examples of Successful Fund Predictions using Machine Learning
One notable example of successful fund prediction using machine learning comes from the asset management firm BlackRock. They leveraged AI algorithms to analyze vast datasets, predicting market trends with impressive accuracy. Their models successfully guided investment decisions that yielded substantial returns.
Another case is Goldman Sachs utilizing machine learning for trading strategies. By employing neural networks, they could identify patterns in historical data, enabling them to forecast price movements effectively.
A start-up called Numerai has also made waves in this space. It crowdsources predictions from data scientists and uses those insights to manage a hedge fund. This unique approach demonstrates how collaborative models can enhance predictive capabilities.
These examples showcase how powerful machine learning can be when applied to real-world financial scenarios, transforming traditional methods into more dynamic and precise forecasting tools.
Ethical Considerations in Using Machine Learning for Fund Prediction
As machine learning becomes a driving force in fund prediction, ethical considerations take center stage. The use of algorithms can inadvertently introduce biases based on historical data. If the input data reflects past inequalities, predictions may reinforce those disparities.
Transparency is another critical concern. Stakeholders need to understand how decisions are made by these models. A black-box approach hinders trust and accountability in financial markets.
Privacy is paramount as well. Handling sensitive financial information requires stringent security measures to protect individuals’ identities and assets from misuse.
Moreover, reliance on automated systems can lead to overconfidence among investors. Human intuition remains essential in navigating complex market dynamics that machines might not fully comprehend.
Ethical frameworks must be established to guide the responsible use of machine learning in finance. Balancing innovation with moral responsibility will shape the future landscape of fund prediction technology.
Future Possibilities and Advancements in the Field of Machine Learning for Fund Prediction
The future of machine learning in fund prediction holds exciting possibilities. As data collection becomes more sophisticated, the algorithms can analyze vast amounts of information in real time.
Enhanced predictive models will likely emerge, incorporating advanced techniques like deep learning. This could lead to more accurate forecasts and smarter investment strategies.
Integrating artificial intelligence with natural language processing may also transform how market sentiment is gauged. By analyzing news articles and social media posts, funds can react swiftly to public perception shifts.
Furthermore, developing explainable AI will make predictions transparent. Investors would gain insights into decision-making processes behind each prediction, fostering trust in automated systems.
Collaboration between financial experts and data scientists will pave the way for innovative tools tailored for specific markets or sectors. The synergy between human intuition and machine efficiency could redefine fund management practices altogether.
Conclusion
Machine learning is transforming the landscape of fund prediction in remarkable ways. As we’ve explored, its applications extend beyond mere calculations; they harness vast amounts of data to reveal insights that traditional methods might overlook. The benefits are clear—higher accuracy, faster processing times, and the ability to adapt to new trends.
Yet, with these advantages come challenges. Data quality issues and algorithmic biases can impact predictions significantly. It’s essential for practitioners in this field to remain vigilant about ethical considerations as well. Transparency and fairness should be at the forefront of any machine learning application used for fund prediction.
Real-life examples highlight the success stories where machine learning has made a tangible difference in predicting market movements or investment opportunities. Companies leveraging these advanced techniques have seen significant returns on their investments.
Looking ahead, advancements in technology promise even more innovative solutions within this realm. As algorithms evolve and become increasingly sophisticated, we can anticipate a future where predictive analytics becomes an indispensable tool for investors.
The journey into machine learning’s role in fund prediction is just beginning but holds immense potential for those willing to embrace it wholeheartedly. Embracing such smart methodologies could redefine investment strategies across various sectors moving forward.
RELATED POSTS
View all